US startup Diskover is a top-down open source metadata management software company with relatively few but significant customers, such as Arm, Disney, Micron and WarnerMedia CNN, and appears to have grown by word of mouth instead of by mass developer adoption. We’ll look at the basic idea behind the company in part one of this examination of Diskover and move on to its AI and other data pipeline activities in a second part. Diskover is a data management company, somewhat like and others.
Its market strategy is to provide the best and widest lens possible through which customers can find, monitor and manage their data estate using direct and derived file and object metadata to set up data pipelines. It has a dozen employees and has gathered a roster of some 60-plus customers, doing so with minimal marketing activity. We were briefed by CEO Will Hall and Chief Product Officer Paul Honrud.
The basic details are that Diskover was founded by initial CEO and current CTO Chris Park in 2016 and developed a web application, to manage files and provide storage analytic functions. It used Elasticsearch for indexing and crawling supported on-premises and public cloud NFS/SMB accessed file systems. The aim was to provide visibility into a heterogeneous data estate, identifying unused files and reducing storage waste.
Diskover has free community edition software and developed supported paid editions – Professional, Enterprise, Media, Life Science – for enterprises. It has also developed a plug-in architecture to make its SW extensible. Paul Honrud became the CEO in June 2021 and Chief Product Officer in July 2024 when Will Hall was appointed the CEO.
Honrud had previously founded and run unstructured data management startup, DataFrameworks, in 2009. Its ClarityNow SW provided data analytics and management functions for file and cloud storage, offering a holistic view of data across heterogeneous storage systems. Dell acquired the company in 2018.
Honrud stayed at Dell as a field CTO for Unstructured File and Object Management until 2020, joining Diskover the next year. Hall, with sales and exec experience at NetApp, Fusion-io and Scality before DataFrameworks, worked at Dell as a global account manager for unstructured data customers but leaving to be VP Sales at wafer-scale company Cerebras in 2020. He then became an operating partner at Eclipse Ventures in 2023, subsequently rejoining Hornrud by becoming Diskover’s CEO the next year.
Diskover says there is a data management spectrum ranging from basic filesystem fault fixers – something goes wrong – to workflow and pipeline operations, where it locates itself. Honrud says the something-goes-wrong suppliers have “a set of data management functionality and it’s clearly targeted to when something goes wrong like a backup or Cohesity; how do I recover when something goes bad, or ransomware? These are kind of like your Veeam, your Cohesity, your RNAs for that matter. Their value really shines when something went wrong.
” Next up, he says: “You have what I call our space tattle tale tools. And this what the traditional storage vendor thinks about space. How old is it? How big is it? When was the last time it was accessed? Are there duplicates? It’s literally a space tattletale tool and that is really hard to build a business off of.
” Space tattle tale tools can be really important: “When the storage is full and they need to go on a crash diet, I immediately need to clean up some stuff, go on a crash diet so I can continue working. Or they buy new storage, they just kicked the can down the road. But you literally in between those time periods can turn the software off and nobody complains.
It is very hard. It’s very hard to build a business model.” The data mobility suppliers are one step on: “If you don’t want to actually change your lifestyle and lead a healthier lifestyle and want to keep going on this crash diet thing, then here you go.
We’ll help you identify where to cut the fat quickly. You have a whole bunch of technologies around data mobility. These are data movers, right? .
.. and there’s like 30 of them.
” “This is a crowded market and there’s more every day. There’s another one called MASV. These are all your companies that are in data movement.
And what they realized with data movement is that it’s hard to build a recurring business. If your data movement is really data migration; they’ve sold a big new Isilon piece of storage and they need to get the data off of old Isilon and onto new Isilon. That is kind of a one-and-done data movement use case.
” Honrud says they moved in a tad: “What they started to realize is that’s not the heart of the problem. The heart of the problem is what data needs to be where, when, to support a workflow. So they started to build analytics, but the analytics are biased because they have a horse in the race.
They’re trying to build analytics that drive data movement, that drive demand to their technology. So look, if you move it over here, it’s cheaper. Oh, look how old this stuff is.
They’re really not understanding the data. They’re just trying to provide analytics to move data.” Now we come to the workflow/pipeline area: “Then you have data management where it comes to workflow and pipeline.
This is what data do I have? Why do I have it and how do I run my business more efficiently?” He says that many organizations are: “generally trying to produce some widget, a movie, a new chip design. They’re trying to cure cancer. And so how do I take the data associated with that and produce more movies shorter, shorten the cycle for chip design? How do I actually manage data to help me run my business better? It’s super hot right now because everybody’s realising if you don’t understand your data and what is good data and bad data, you have no way to feed your AI models.
” Diskover’s software scans and indexes data, builds a metadata catalog, and uses that to support searches, analysis, actions and automation: Its software consists of scanners and ingesters to populate the catalog, elasticsearch to support management tools for finding data amongst files and objects, analyzing the data, orchestrating it and visualizing it. The software supports Parquet for feeding data to data lakes and lakehouses: It’s built to operate in a scale-out environment assuming a distributed index of storage repositories, and with a scale-out index. Honrud says its continuous, cached and parallel scanning can connect to any file system and handles massive amounts of data: The scanning technology uses both direct, system-produced file and object metadata, and deduced indirect metadata.
Honrud talks about reverse engineering access fingerprints left on data. He said: ”If I came over and looked in your garage or I came over and looked on your laptop, I could tell whether you like tennis or bicycling or stamp collecting. I could tell a lot about you by the fingerprint there.
” “That’s the first thing. Second thing is most storage vendors, and most people in data management try to manage data at the end of the food chain. When it’s time to archive it.
Oh, it’s over three years old. Let’s move it. The whole thought processes around there, it is way too late.
“You’ve missed way too many opportunities to capture metadata, but the content is most valuable when it’s originally created, when it’s coming off of a camera or a microscope or a genome sequencer. So let’s move our thought process for data management and metadata to when data originally lands on the storage and then let’s follow that data through its lifecycle. Okay.
“So when a new piece of storage is provisioned out, it usually gets provisioned by somebody creating a service ticket in Jira or ServiceNow. That ticket goes to the IT department, they create an NFS share, an SMB share, and an S3 bucket. And then they close the ticket and the business community is now allowed to start putting data on storage.
First time they missed an opportunity to capture metadata, right? Because in that Jira ticket is usually the project name, project owner, project start date, and probably estimated project end date. But if you just create the share and leave, you just dropped a bunch of metadata on the floor.” Diskover picks up that discarded potential metadata and uses it.
There’s more to say about this though. Honrud adds: “The next way to capture metadata, yes, is if the owner and group are actually accurate on the file system. In other words, I may be the project owner, but you were the guy that loaded the data on the file system.
So you show as the owner, but I’m the guy that’s actually running the whole project. So first thing that you miss there is you’re talking to the wrong guy. Like, Hey Chris, what should we do with this data? Well, you’re not the guy using it.
You’re the guy that loaded it. So you need to know project owner, project primary investigator in a research environment. That information you can find if the user logs in via AD or LDAP.
You could do a reverse lookup in Active Directory or LDAP. There’s a lot of metadata there. I could usually tell who you report to, what division you are in.
” Diskover scans and indexes the data and ingests the index metadata into Elasticsearch. It can then feed it upstream to visualizers, data warehouses and lakehouses and so forth. In part two of this look at Diskover we’ll examine its upstream pipeline supporting capabilities.
.
Technology
Discovering Diskover’s data management discipline
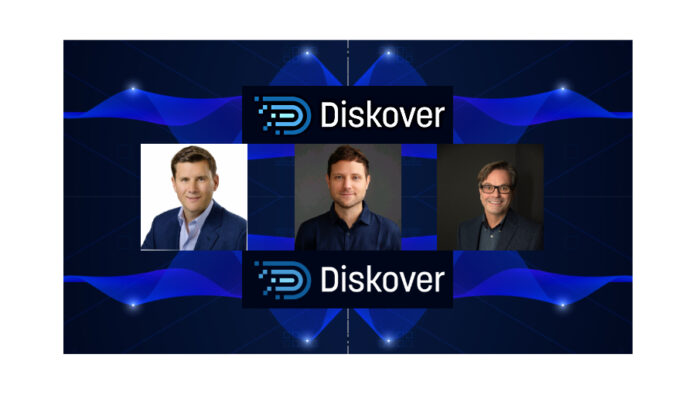
US startup Diskover is a top-down open source metadata management software company with relatively few but significant customers, such as Arm, Disney, Micron and WarnerMedia CNN, and appears to have grown by word of mouth instead of by mass developer adoption. We’ll look at the basic idea behind the company in part one of this [...]