Created Using GPT-4oFoundation models have redefined what AI systems can do by being pretrained on vast, diverse datasets across text, images, and multimodal content. However, sourcing high-quality, real-world data at this scale poses major constraints in terms of cost, coverage, and control. Synthetic data—artificially generated through simulations, generative models, or programmatic logic—has emerged as a compelling alternative or complement for both pretraining and post-training.
This essay explores synthetic data's role in training foundation models, presenting core arguments for and against its use. It spans application domains like vision, NLP, and robotics, discusses real-world case studies, and reviews the dominant techniques for generating synthetic data. Finally, it evaluates where synthetic data excels and where it falls short, offering a framework for its effective use in large-scale AI pipelines.
Benefits of Synthetic Data for Foundation Models Read more.
Technology
The Sequence Opinion #529: An Honest Debate About Synthetic Data for Foundation Model Training
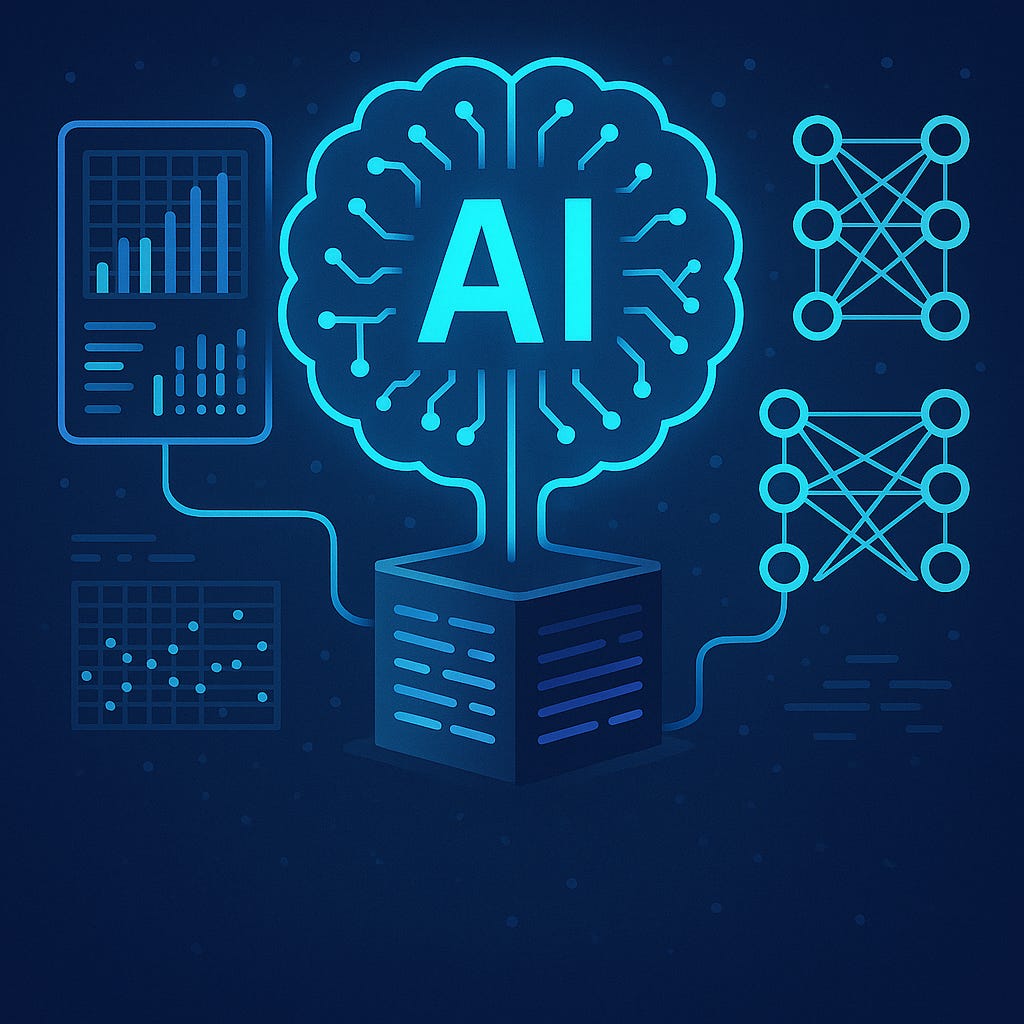
Values, challenges and applications of one of the next frontiers in generative AI.