As the AI and data science arena continuously evolves at a tremendous pace, technology is revolutionizing how we think about residential safety and efficiency. One of the advancements is from data scientist Paril Ghori, who has effectively used an Autoencoder deep learning model to identify anomalies in residential furnaces. Utilizing cutting-edge machine learning, innovation focuses on residential safety while reducing the risks involved with faulty appliances.
Paril Ghori, a renowned expert in data science and AI analytics, has an experience range of deep learning, big data processing, and predictive analytics for applying high safety and operation efficiency in home automation systems. His efforts and strategies have played a pivotal role in leading the way toward innovative AI applications to minimize risks, especially in home appliances. The expert claims that “deep learning system identifies anomalies in high-frequency amperage signals from household furnaces in real time, which could signal malfunctions.
” Through the use of PySpark, Pandas, and Databricks, he optimized data processing rate by 50%, making it possible to analyze large-scale furnace data almost instantaneously. This game-changing technology can potentially cut response times for detecting irregularities by 40%, greatly enhancing safety controls and preventing hazards from faulty heating systems. Reportedly, the solutions devised by him, led to a 30% decline in service interruptions and maintenance costs by proactively detecting and addressing furnace irregularities before they escalate.
“These improvements highlight the profound impact that AI and deep learning can have on residential safety and risk management”, says Paril. He built a multivariate time series forecast model based on random forest regression to forecast electricity usage, lowering prediction errors and improving grid reliability. “I created an end-to-end data pipeline with Databricks for smooth integration, model training, and deployment of large-scale AI applications”, he comments.
During the development of this anomaly detection system, he resolved quite a number of significant challenges. Among the most important was how to process high-frequency data streams from furnace amperage signals. With PySpark as a means of distributed computing, he made it scalable and efficient to process huge volumes of operational data.
He has also partaken in the research domain. The research highlights a larger industry trend, that is, the growing uptake of AI-based anomaly detection systems among home automation. As smart home technology advances, real-time monitoring of run-time data is increasingly becoming an imperative aspect of maintaining residential security.
His vision for the future of AI-based safety solutions reinforces the need to combine anomaly detection with Internet-of-Things -enabled devices, enabling predictive maintenance and proactive risk avoidance. In spite of the revolutionary approach of his work, he continues to be geared towards ongoing innovation. In the future, he envisions that AI-based safety systems will be a standard fixture in every home, smoothly embedded with IoT technology to provide active maintenance and prevention of hazards.
With experts like Paril Ghori at the forefront, the integration of AI and home security is poised to revolutionize how we engage with and protect our homes. As the future of AI-fueled safety solutions grows closer, Paril's work represents the potential power of data science to revolutionize daily life. His anomaly-detection work with home furnaces is only a start to what will be an extensive revolution for smart home innovation, where artificial intelligence not just makes life easier but also improves the safety and health of countless residents across the globe.
.
Business
Data Scientist Implements Autoencoder Deep Learning Model for Detecting Anomalies in Home Furnaces, Prioritizing Safety and Minimize Risk
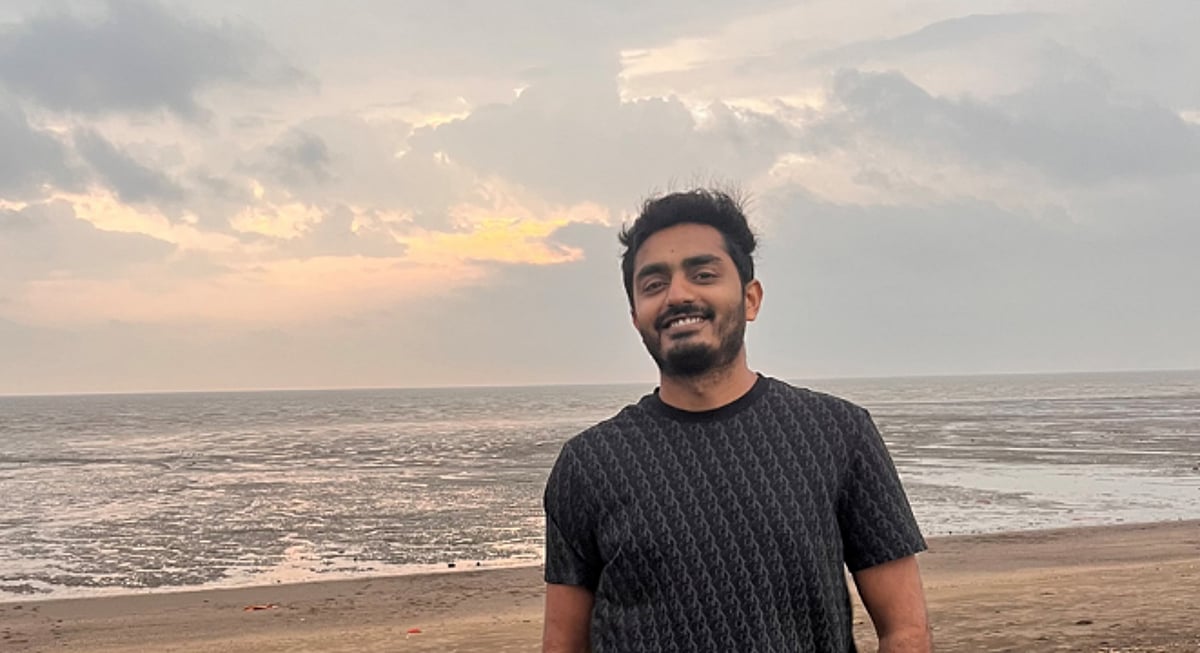