By Anurag Sanghai, Principal Solutions Architect, Intellicus Technologies In 2025, directors and CEOs will witness their AI-powered data assistant determine market shifts before they happen, reveal patterns invisible to human team members and guide critical decisions with accuracy. This is the reality generative AI has brought to data analytics. With the convergence of GenAI and cloud computing, the field of data analytics is making significant strides enabling businesses to derive higher value from data than ever before.
If the past decade witnessed traditional data analytics evolving from descriptive to predictive analytics, the next era is seeing generative analytics create real-life scenarios of what could happen in future. But that is not all. Cloud platforms have further fueled the use of GenAI by providing computing power, scalability and flexibility needed to deploy AI-powered analytics.
How Generative AI Is a Game-Changer for Data Analytics Generative AI has led to a massive shift in the field of data and analytics where human analysts and AI team up to produce data stories, uncover valuable insights and drive data-informed decisions. Data analytics, powered by AI and machine learning (ML), goes beyond automating tasks such as cleaning large data sets, management of data pipelines or basic analysis. Today, GenAI also facilitates more valuable actions such as model interpretation or problem visualisation.
Gen AI has also enhanced predictive analytics by adding several layers of capabilities. AI algorithms built on top of data analytics models can handle complex and nonlinear relationships in data, adapt to changing conditions and can even identify subtle patterns leading to precision in predictions. Natural language interfaces (NLIs) such as chatbots and AI assistants have significantly reduced technical skills needed to query data.
Nontechnical users can now interact with data in business language, encouraging deeper data exploration and broader collaboration between teams. By leveraging ML algorithms, GenAI analyses data, identifies patterns, creates visual outputs and summaries in simple language. Through its ML models, it also learns from an organisation’s existing data to create new synthetic datasets that closely mimic the original.
This functionality lets businesses create large datasets for testing and experimenting, without worrying about privacy and data regulations. Gartner estimates that by 2030, synthetic data will dominate real data in AI models empowering businesses to produce a significant number of real-world scenarios. The Cloud’s Role in Scaling Generative AI Cloud platforms such as Azure or AWS offer unparalleled computational resources that AI models need to process substantial data for elaborate tasks.
Their CPUs (Central Processing Units) and GPUs (Graphics Processing Units) help in accelerating processing for GenAI to create simulations and expedite model training and iterations. Training and deploying generative AI models in data analytics environments need substantial amounts of processing power, storage and memory, along with increased computational demands. The cloud provides dynamic and scalable infrastructure while seamlessly adjusting resources for generative AI to manage large data clusters and handle increased user interactions.
Another critical factor for the success of GenAI is the availability of structured and unstructured data in the form of texts, images, videos, audio files, feedback, comments, social media posts etc. Cloud ecosystems unify large amounts of such data and provide a playground for GenAI to experiment, process and get training data for future analyses. How is Cloud GenAI Finding Relevance in The Real World? Today, ecommerce companies offer dynamic marketing content on their homepage where buyers see personalised banners based on their search history.
Similarly, AI models have hyper personalised product recommendations based on buyer’s preferences in terms of prices, fitness goals, interest in certain brands, frequent buying of certain types of products and even delivery time. Most online stores have chatbots that not only answer queries but also recommend options tailored to the customer’s needs. Another use case is found in the field of finance where financial services firms use Generative AI models hosted on the cloud to simulate possible (what if) scenarios such as: What if 20% of our auto-loan customers delay payments for three months? AI models generate synthetic customer behavior datasets based on similar past challenges (like COVID-19 or 2008 stock market crash) and produce multiple narratives along with estimated financial impacts.
Cloud-based computing aids in this process as it scales to run thousands of simulations in parallel while AI models recommend future actions such as refining risk scoring models, adjusting lending criteria or even creating new short-term liquidity products for vulnerable customers. Cloud-based GenAI also facilitates automated data cleaning and augmentation. This capability allows users to identify inconsistent, incomplete or duplicate data, freeze or block such data from further processing and even fill in gaps in datasets when data is missing.
The most business-user-friendly advancement, however, is in the field of conversational analytics. AI’s ground-breaking generative abilities have taken self-service analytics to a whole new level as it empowers lay persons to use easy voice or text prompts for deriving real-time predictions and forecasts, without any technical expertise. A Word of Caution While GenAI with cloud promises exceptional capabilities, it does come with its share of drawbacks.
One of the biggest concerns is propagation of bias. If the training data has biases, AI will most likely replicate and even amplify those biases, creating inaccurate and inconsistent results. Apart from accuracy concerns, GenAI cannot completely rule out the possibility of privacy breaches if the model is used without security protocols.
When GenAI is used with cloud platforms, there’s an increased need for monitoring. Since cloud provides unprecedented infrastructure access to GenAI for processing data, workloads can spiral quickly, leading to escalating costs. Despite these limitations, cloud-driven generative AI has shown capabilities that enhance the field of data analytics and increase business competence for organisations across industry verticals.
The combination is not only progressive or incremental, but it has transformed analytics by facilitating accessibility combined with creative narratives, speed and accuracy. if (!window.AdButler){(function(){var s = document.
createElement("script"); s.async = true; s.type = "text/javascript";s.
src = 'https://servedbyadbutler.com/app.js';var n = document.
getElementsByTagName("script")[0]; n.parentNode.insertBefore(s, n);}());} var AdButler = AdButler || {}; AdButler.
ads = AdButler.ads || [];var abkw = window.abkw || '';var plc516228 = window.
plc516228 || 0;document.write('');AdButler.ads.
push({handler: function(opt){ AdButler.register(182450, 516228, [728,90], 'placement_516228_'+opt.place, opt); }, opt: { place: plc516228++, keywords: abkw, domain: 'servedbyadbutler.
com', click:'CLICK_MACRO_PLACEHOLDER' }});.
Top
Generative AI and the Cloud: The Next Big Leap in Data Analytics
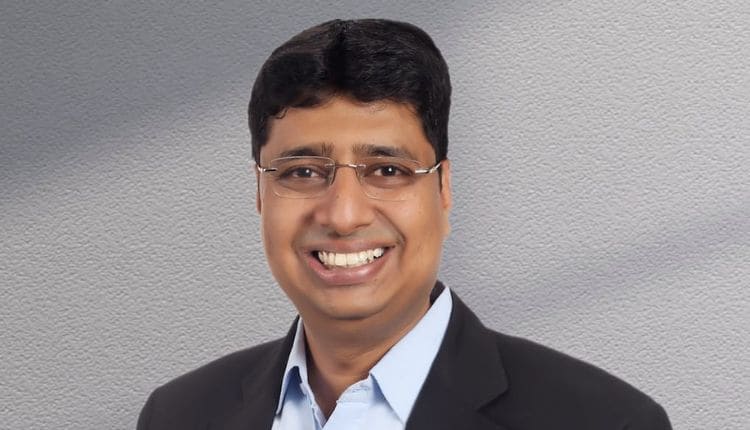
In 2025, directors and CEOs will witness their AI-powered data assistant determine market shifts before they happen, reveal patterns invisible to human team members and guide critical decisions with accuracy. This is the reality generative AI has brought to data analytics. With the convergence of GenAI and cloud computing, the field of data analytics is making significant strides enabling businesses to derive higher value from data than ever before.The post Generative AI and the Cloud: The Next Big Leap in Data Analytics appeared first on Express Computer.